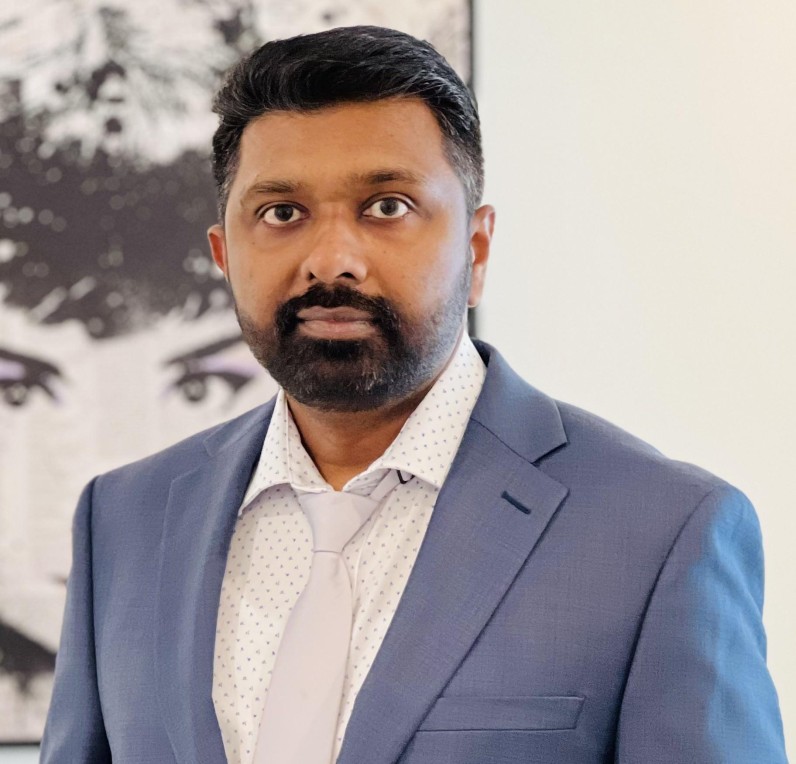
Artificial intelligence is empowering leaders in various industries to make smarter decisions and personalize customer experiences. But in healthcare, it's changing the way medical professionals treat their patients and prevent illnesses. Today, tools like machine learning are helping doctors analyze patient data, catch diseases sooner, give personalized health tips, and even improve insurance ratings.
At the forefront of this initiative are experts like Ginoop Chennekkattu Markose, senior engineer lead at a leading health insurer. As he's developing tools in predictive analytics, data warehousing, cloud technology, and chronic disease detection, he's helping doctors offer more targeted and effective care worldwide.
How Ginoop Is Shaping Healthcare with Predictive Analytics
Ginoop Chennekkattu Markose has long had a knack for data and problem-solving. After graduating with a master's in computer applications, he worked as a systems engineer before taking a leadership position at a multinational information technology services and consulting company. There, his work was primarily in data warehousing: organizing and storing massive amounts of information so it's easy to access, making sure systems can handle complex data tasks.
However, Ginoop soon made another career shift to healthcare, where he saw firsthand how data had the power to improve lives. Specifically, he found that predictive analytics can be used to analyze current patient health data and identify precursors for chronic conditions like diabetes, heart disease, and COPD.
As lead solution architect at a leading health insurer, Ginoop built a platform that accomplishes exactly this. So, instead of waiting for symptoms to show up (at which point the disease may already have progressed too far), doctors can now be alerted to these risk factors sooner and develop personalized care plans to prevent disease progression. This not only helps patients avoid severe complications but also reduces the need for expensive treatments down the line.
Ginoop published his findings in a paper titled "Machine Learning Algorithms for Early Detection of Chronic Diseases in the Medicare Population," detailing several techniques that he relies on to predict chronic diseases: support vector machines, random forest algorithms, and neural networks.
Support Vector Machines
Support vector machines (SVMs) are machine learning models that serve various purposes but are commonly used to sort data into categories precisely. In healthcare, they can look at vast sets of information (like patient demographics and clinical records) and try to delineate a clear line that divides the data into different categories—in this case, separating patients at high risk from those at low risk.
"SVMs have been shown to be highly accurate in spotting early signs of diseases by analyzing patient data," Ginoop says. "However, they do have some drawbacks: for example, they require a lot of computer power to handle big datasets, and they work best when data can be separated neatly (which doesn't always happen in real-world medical data)."
Random Forest Algorithms
Random forest algorithms have a similar purpose as SVMs, but they go about it in a very different way. They work by creating a vast number of decision trees (like multiple "if-then" statements), evaluating each data point, and progressing down each tree until it reaches the most logical conclusion. Its prediction then becomes the most common conclusion out of the hundreds to thousands of decision trees it evaluated. This approach is particularly useful in healthcare, where there are often lots of different factors in patient data.
"Random forests are also good at handling missing data, which is common in healthcare," Ginoop explains. "For instance, a patient's record might have gaps if certain tests weren't done, or if certain symptoms weren't reported. While some models struggle with these gaps, random forests can still make reliable predictions by using the available information across multiple decision trees."
Neural Networks
Neural networks are a type of machine learning model that mimics the pattern recognition and problem-solving capabilities of the human brain. Just like the brain uses connections between neurons to process information, neural networks use layers of interconnected nodes to analyze patterns. This ability to find complex, nonlinear patterns makes them particularly useful for predicting diseases with multiple contributing factors, like COPD or heart failure.
However, they require large datasets and a lot of computational power, making them challenging to implement in practical clinical settings. As a result, they're more often used in research or larger healthcare systems that have the resources needed to support them.
Algorithmic Bias and the Need for Diverse Data
While these tools are powerful, they can still fail to make accurate predictions if the data used to train them isn't representative of all patient groups. This is known as algorithmic bias.
For example, if data from specific Medicare subgroups (such as certain age groups or ethnicities) is missing, the model may struggle to predict health outcomes accurately for those patients.
"To reduce this risk," Ginoop explains, "it's crucial to use a diverse and representative dataset that reflects all of the populations that the model will serve. A well-rounded dataset helps these algorithms make accurate predictions across varying groups, leading to fairer, more effective healthcare outcomes for everyone."
Expanding Preventive Care with Cloud Scalability
Cloud platforms like AWS and Snowflake make it easier to access, share, and synchronize real-time data—a crucial need in vital industries like healthcare. So to make his predictive analytics platform even more accessible, Ginoop led the migration of important datasets to these cloud platforms. Now, healthcare professionals can use this platform to perform self-service analytics—analyzing patient data and pulling insights themselves without having to rely solely on data specialists.
"Moving to the cloud," Ginoop notes, "means quick, informed decisions are more accessible than ever."
How Ginoop's Data Storage Approach Drives Better Healthcare Decisions
However, even with a vast amount of data available, it's not much good if it's not stored and organized properly.
To this end, Ginoop led the creation of a data warehouse—a centralized system that brings together data from various sources. To simplify the process and automate what would have been countless hours of menial labor, he built automated frameworks that could quickly sort, verify, and organize incoming data.
A standout feature of this workflow that Ginoop oversaw the implementation of was a real-time medication adherence framework that tracks whether patients are regularly taking their prescribed medications. By spotting any gaps or inconsistencies, providers can step in sooner to work with patients on their treatment plans.
This data storage framework has helped improve patient outcomes by giving healthcare teams accurate, up-to-date data to guide their decisions. It's also helped Medicare programs improve their star ratings—scores that reflect the quality of care and influence funding.
Ginoop Chennekkattu Markose: Catching Health Issues Early with AI-Driven Care
Ginoop's contributions haven't gone unnoticed. In recognition of his work, he earned a 2024 Global Recognition Award and was named by the Titan Health Awards as a Healthcare Analytics Expert.
Today, Ginoop continues to drive practical, data-driven solutions across the healthcare industry. He imagines a future where AI takes healthcare even further, with machine learning built directly into electronic health records to give doctors instant insights for on-demand, personalized care.
To learn more about the benefits of machine learning for preventive care, follow Ginoop on Academia.
Join the Conversation